25.11.2022
How the science and technology company is looking to speed up the way new drugs get developed and approved
Clinical trials are an important step in getting new pharmaceuticals to market, but they are complex and rely on multiple interdependent factors. This makes it hard to plan them optimally. Attempts to optimize trial designs with conventional machine learning are hitting their limits. That’s why science and technology company Merck is testing the use of Bayesian networks and quantum computers – and reaching out to other experts in QUTAC.
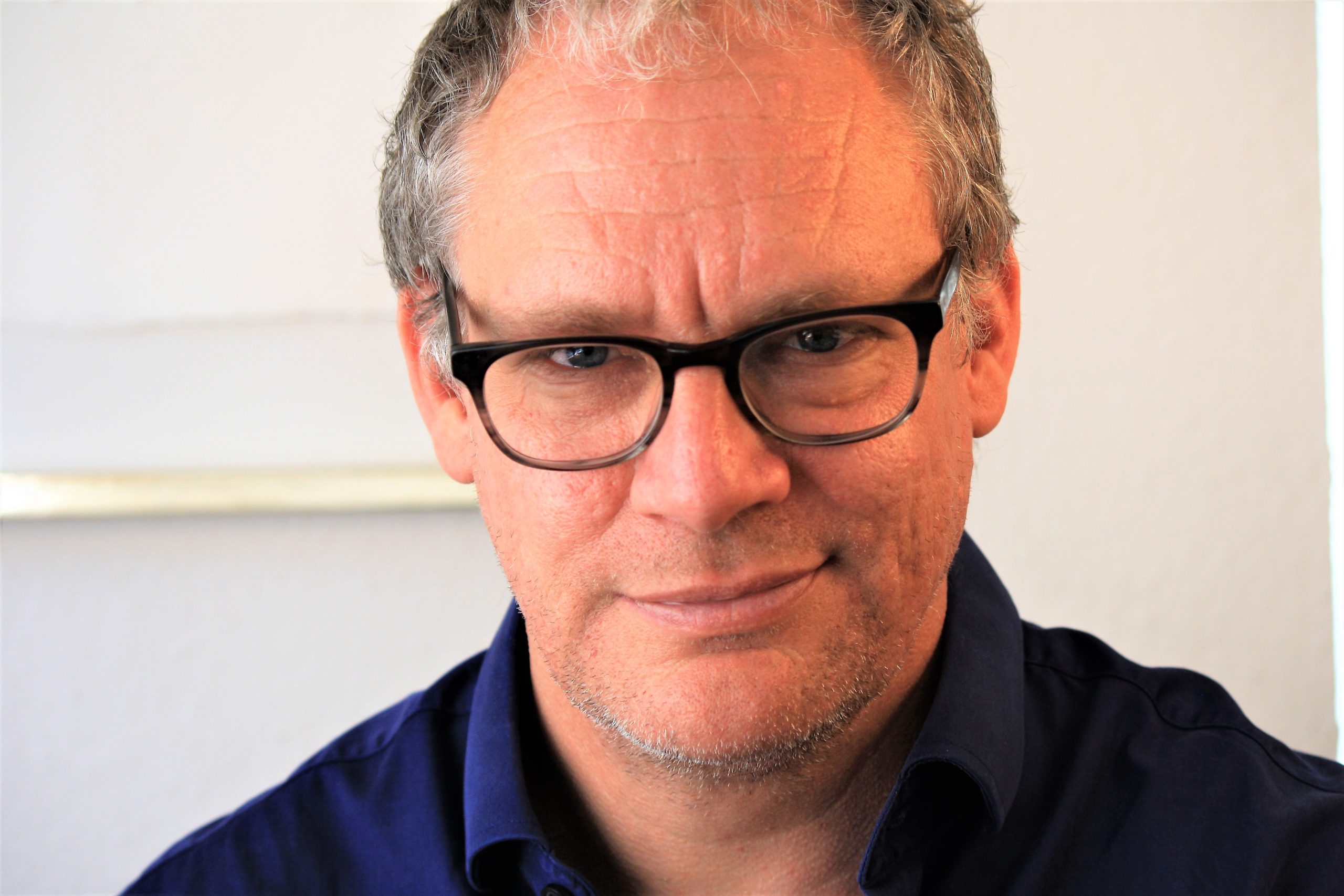
Thomas Ehmer, Innovation Incubator Lead at Merck © Merck KGaA
Planning clinical trials: a complex challenge
The fact that multiple coronavirus vaccines were developed within a relatively short time – just over two years – is considered a milestone in pharmaceutical research. “In the bigger scheme of things, though, this kind of success is still the exception”, explains Thomas Ehmer. “Usually, development and approval for new drugs takes significantly longer.” One major reason is the need to conduct clinical trials to test the new product’s efficacy and safety. There are many factors affecting how long the trial takes and how economically successful it is. That’s something Ehmer, Innovation Incubator Lead at the Merck Group, knows all too well: he and his colleagues work intensively on innovative solutions to improve clinical trials, such as by making them more efficient.
One example Ehmer gives of how complex these trials are to plan is coordinating participant recruitment and producing the necessary medication. “Ultimately, it’s all a matter of timing. If you are too ambitious and recruit a large number of participants early on, it gets difficult and expensive to provide enough medicine in time, especially when you’re talking about biologics. If planned recruitment is delayed, on the other hand, drug compounds might end up unusable because of their shelf life.” Pharmaceutical products, too, do have expiry dates. Uncertainties like these mean that companies often fail to pull their weight when it comes to clinical trials. “48 per cent of trial facilities miss their recruitment targets. 75 per cent of trial protocols end up needing adjustment,” as scientific surveys point out, so one thing is certain for Ehmer: “optimizing clinical trial planning not only benefits the pharmaceutical industry financially. First and foremost, it actually helps patients, who don’t have to wait as long for new drugs to be approved.”
Optimizing clinical trials through Bayesian networks and quantum computing
Currently, pharmaceutical and biotech companies use digital planning tools to get better estimates of the different effects certain factors will have on how their clinical trial studies play out. Merck employs a simulation platform called ODeSA (Operational Design and Study Accelerator), which takes inputs representing various key elements and calculates the most likely recruitment curve for the study – the way the expected participant numbers will evolve within a certain timeframe. This system allows the company to run various recruitment scenarios. As Ehmer cautions, however, “so far the process remains a very iterative one.” Merck’s experts run suggestions for a study design through ODeSA which calculates scenarios based on those parameters. “Either the results manage to satisfy us – or they don’t. In the latter case, we adjust our combination of inputs until we generate a better recruitment curve.” The team repeats this process until it gets an optimal preliminary result. “There are things the system can’t do just yet, however: optimize the study design in an automated way, in other words tell us what the key factors need to look like to have an ideal recruitment curve – the reverse of our current procedure. Such optimization problems are simply too complex for applications running on conventional computers.”
That is why Ehmer’s team is exploring the possibilities quantum computing may bring to the field. In a project funded by the German Federal Ministry of Education and Research, Merck is working with partners to design, develop and assess quantum algorithms that can be used to optimize clinical trials. A major component of this project is the usability of Bayesian Networks. These are probabilistic graphical models that allow a number of variables and their conditional dependencies to be represented graphically. They are highly suited for determining the probabilities by which a particular real-life event’s occurrence can be attributed to various possible known causes. When it comes to determining the ideal inputs for an optimized clinical trial study design, such networks seem to be a natural fit. Conventional computers, nonetheless, are increasingly running up against their limits when asked to work with networks as complex as these. Quantum computers could well be the answer.
QUTAC: Cross-industry collaboration and innovation
The project also aims to find out if this kind of optimization is solvable with today’s generation of quantum computers, or those of the near future, in the context of the NISQ era (“Noisy Intermediate-Scale Quantum”). Thomas Ehmer is optimistic and sees the project as having major significance if it succeeds, over and above the clinical trials use case: “if we can show that quantum computers work in practice for optimizing complex dependency relationships using Bayesian networks, it will allow us to tackle a raft of similarly complex challenges” – such as CO2 emissions reductions and better containment of infectious diseases. Emissions and disease are phenomena that are frequently driven by a similarly opaque set of interacting factors, Ehmer says, and in both fields there is so far little or no data available on many of the factors and their relationships, rendering it almost impossible to employ either data-hungry classical machine learning or classical simulations. Emissions and disease are thus prime candidates to be analyzed, simulated and optimized using Bayesian networks. “Ideally, in the future, we’ll have the system suggest different possible solutions, which we’ll just need to check for reasonableness when they come out.” This could lead to new correlations being found, specific solutions emerging for the problem of non-existent data, and ultimately the opportunity to use conventional methods to re-validate predictions made by the system. “A win-win achieved by skillfully varying new and existing methods.”
The project’s progress has greatly benefited from the Merck team’s contacts with colleagues outside, especially via QUTAC, says Ehmer. “The Consortium is one of the few places where experts with similar backgrounds and similar challenges can come together and discuss their approaches. Everyone is working on solutions that have to function in large industrial company contexts. On the other hand, our industry-specific frameworks are sometimes very different and the people behind QUTAC are highly diverse. There are no blinkers, there is no silo thinking. Just the will to innovate.”